Listen to the article – The Transformative Effects of AI on Business Operations
Key takeaways
- AI implementation enhances business efficiency, accuracy, and decision-making, leading to improved overall performance and competitive advantage.
- Transition to AI-driven processes likely brings risks, including implementation, operational, financial, human capital, and ethical risks, all of which potentially affect the business operations, stability and profitability of the organizations.
- Regulatory compliance of AI is evolving rapidly, and various regions are taking steps to address the risks associated with it. The EU, UK and US are a step ahead in regulating the development and use of AI.
- Relying on external AI providers introduces risks such as vendor lock-in, innovation dependency, intellectual property issues, service interruptions, data ownership and security concerns, and potential shifts in the vendor’s business model.
01
Foreword
01
Foreword
Artificial Intelligence, which is programmed to complement human intelligence and exerts considerable influence on the corporate world. The rapid integration of AI into business operations marks a critical shift of how companies operate in the global market.
Today AI is prevalent in various software applications, revolutionizing workflows and business practices, and organizations have already noted the material benefits of utilizing AI tools.[01] This technology is also being used in various industry segments, such as marketing and sales, operations and supply chain, finance and accounting, human capital, legal, defense, cybersecurity and many more. In fact, AI technology is improving customer engagement and experience chatbots, call bots and assistants, that revolutionized customer service interactions and provided efficient avenues for client engagement. Moreover, data analysis capabilities of AI technology enable processing of large amounts of data, providing insights, recognizing patterns, making conclusions and predictions.
How can we help?
Intelligence Solutions
The combination of business, market and strategic intelligence ensures result-driven outcomes for our customers.
Risk management
Risk management through the responsibility of taking risk ownership while ensuring safety and security
AI technology enhances the efficiency if existing software tools by automating repetitive tasks, such as data entry, meeting notes and content generation.[02] Also, AI can identify customers’ preferences and purchasing behavior by analyzing big consumer data, which further enables businesses to offer personalized recommendations and target specific audiences.[03] Moreover, AI implementation proposes integrating AI technologies into a business’s operations and its processes, and triggers decision-making to enhance efficiency, predictability, accuracy, and overall performance. This implementation utilizes computer software (learning machines) capable of performing tasks like human learning, planning, and problem-solving. All the above AI abilities empower businesses to make strategic and operational decisions about customers, product offerings, growth and their future business directions.
While the benefits of AI are clear, the transition path of its applicability highly likely is challenging and risky. This report discovers the AI transition risks, following the regulatory landscape. Additionally, the report presents the risks and potential consequences of relying on external AI vendors. The report seeks to help stakeholders in understanding the risks associated with AI-driven business transformation.
Risk | Risk Factors | Mitigation |
Implementational Risks | Complexity of integration, change management and data quality issues | Identify and evaluate risks, develop mitigation strategies, implement governance frameworks, document and review, continuous monitoring, technical evaluation of data quality, accuracy, robustness and stakeholder inclusion |
Operational Risks | Downtime, over-reliance on AI, algorithmic bias | Identify and evaluate risks, develop mitigation strategies, continuous monitoring of emerging risks, assessing potential bias, documentation and review |
Financial Risks | Cost consideration, risky ROI and market volatility | Identify and evaluate risks, develop mitigation strategies, geopolitical assessments, continuous monitoring, documentation and review |
Human Capital Risks | Talent shortage, workforce displacement and outdated training programs | Identify and evaluate risks, develop mitigation strategies, pre-employment background checks, profiling, OSINT, media monitoring and analysis |
Ethical Risks | Data privacy, transparency | Identify and evaluate risks, develop mitigation strategies, continuous monitoring, ensuring fairness, maintain transparency in AI decision-making, documentation and review |
Regulatory Compliance Risks | Evolving regulations, various jurisdictions and domain issues | Review and analysis of relevant legal and regulatory requirements, data protection laws, industry-specific regulations, continuous monitoring, ethical guidelines, documentation and review |
02
Discovering AI transition risks
02
Discovering AI transition risks
Transition to AI-driven processes likely brings risks, including implementation, operational, financial, human capital, and ethical risks, all of which potentially affect the business operations, stability and profitability of the organizations.
- Implementation risks encompass the complexity of integration, change management concerns, and data quality issues.
Complexity of integration refers to the technological and operational challenges when embedding AI solutions into existing business processes and infrastructure which is a complex and time-consuming effort. In addition, AI technologies require sophisticated hardware and software setups, and these challenges amplify if the existing infrastructure is outdated or incomplete.[04] Another consideration is the fact that AI models, if not properly calibrated or aligned with the company’s business objectives can produce inaccurate outputs or even fail to deliver the anticipated improvements in efficiency. Beyond technological challenges, integrating AI solutions with existing systems involves crossing organizational complexities and cultural barriers. On the other hand, siloed departments, competing priorities, and resistance to change can impede collaboration and coordination, delaying the integration of AI into business processes.
As a result, businesses will likely face delays and unexpected technical issues that bear the potential to disrupt operations and business continuity.
Change management as a transition risk will likely impact organizations in multiple ways, driven by diverse objectives. Integration of AI technologies will highly likely enhance current management processes, boosting efficiency and helping both organizations and their employees perform better. Additionally, some changes will be transformational, prompting organizations to rethink their operations, services, and value propositions. These changes targeting efficiency and growth.
Therefore, transitioning into AI will adjust in workflows, roles, and responsibilities within the organization. Resistance to these changes by employees and management will likely impede the transition process and lead to delays and inefficiencies.[05]
Download Report
The Transformative Effects of AI on Business Operations
AI implementation enhances business efficiency, accuracy, and decision-making, leading to improved overall performance and competitive advantage.
Moreover, ineffective change management strategies introduce the risk of incomplete utilization of AI capabilities and would likely result in suboptimal performance and poor return on investment.
Data quality issues present critical risks to the effectiveness of AI systems, as poor data quality can lead to incorrect or misleading information.[06] AI systems rely heavily on large amounts of data to function effectively, and the quality of this data has a direct impact on the accuracy and reliability of outputs. In fact, these so-called “hallucinations”,[07] are a fundamental issue associated with AI technology and remains unresolved for the time being. Therefore, if the training data input is biased, the AI model will likely produce biased and/or inaccurate results, which lead to unfair treatment of certain demographics. Inconsistent or “noisy” data degrades the performance of AI models. So, models trained on such data may struggle to learn meaningful patterns, resulting in lower accuracy and effectiveness.
Therefore, poor data quality can lead to increased costs for organizations. This includes the costs associated with correcting errors, retraining models, and addressing the consequences of incorrect predictions. Additionally, poor data quality can lead to lost opportunities and reduced customer trust. Besides, inaccurate data output from the AI models leads to regulatory and compliance issues. As a result, organizations may possibly face legal consequences if their AI systems produce discriminatory or incorrect outcomes or violate data protection regulations.
- Operational risks for organizations include downtime, over-reliance on AI, and algorithmic bias, which becomes evident once AI is integrated into business processes, affecting functionality and reliability.
Downtime associated with maintenance, technical issues or cybersecurity breaches could impact business operations and profitability of the organizations. Like any other complex software, AI technologies require maintenance and updates for optimal functionality.[08] Regular maintenance usually leads to system downtime, during which business processes will likely be interrupted. Additionally, unexpected technical issues can cause unplanned downtime, which has the potential to create productivity losses, missed opportunities and financial repercussions. In addition, cybersecurity threats are increasingly sophisticated with generative AI, broadening the specter of malicious activities, and introduces new risks by enabling cybercriminals to deploy sophisticated attacks with greater ease and precision, which have the potential to overwhelm traditional security measures and prolong the downtime of the implemented AI system.
Thus, system downtime due to cybersecurity attacks introduces new risks that traditional security measures are not capable of addressing.
Over-reliance on AI poses another operational risk, where companies and employees become excessively dependent on AI systems for projects management and decision-making and process management. Such over-reliance on AI can result in undermining creativity, ethical judgment, neglect of human oversight and critical thinking,[09] potentially compromising content-creation and decision-making quality, which could well lead to significant disruptions of the overall business operations. In fact, excessive dependence on AI diminishes human touch, resulting in generic outputs and miscommunication.[10] Moreover, insufficient human oversight of AI systems can exacerbate errors and lead to overconfidence in AI-generated outputs, degrading the quality and relevance of AI-generated content.[11]
Hence, over-reliance on AI undermines human creativity, critical thinking and ethical judgment, which further erodes content creation and decision-making quality. Leaving humans out of the loop could additionally result in generic outputs, lower quality and relevance of products, creating risks that directly affect organizations stability and profitability.
Algorithmic bias can lead to unfairly allocated opportunities, resources, or information, infringing on civil liberties and putting individuals’ safety at risk. AI systems are trained on big data which more than often contains biases, whether due to historical inequalities, demographic imbalances, or simply flawed data collection methods. Over-relying on AI for content generation can lead to perpetuating biases which are present in the training data input and can result in inaccurate outputs. It can result in a failure to provide the same quality of service to everyone, negatively impacting people’s well-being through derogatory or offensive experiences.[12] Additionally, it can cause internal conflicts and prompt employees to demand more ethical practices within the organization. Thus, the AI model that produces harmful projections,[13] have the potential to damage organizational reputation, customer trust and the profitability.
Therefore, algorithmic bias deriving from flawed or poor-quality training data input can damage business reputation and customer trust, additionally exerting negative impact to businesses’ operations and profitability.
- Financial risks while adopting AI solutions include cost considerations, uncertainty about return on investment, and market volatility. Together, these factors can affect an organization’s long-term stability and profitability.
Cost considerations in the form of upfront expenses, licensing, infrastructure and implementation or integrating will likely strain budgets and lead to deficit, should the AI project underperform. In addition, continuous operational costs involve expenses such as system maintenance, model updates, and energy consumption, will also exert pressure on profit margins and affect cash flow. Another consideration in this context are the costs associated with human capital and data acquisition.[14] Hiring skilled AI professionals or upskilling existing staff can be expensive.[15] Moreover, data acquisition is a time and cost intensive process required to collect, cleanse and annotate data to remove eventual inconsistencies and errors, for the data to be eligible for training purposes of AI systems.[16] Additionally, safeguarding data and compliance with regulations counts to the operating expenses. Thus, adoption of AI-inclusive business processes must include financial considerations related to planned and hidden costs.
The return on investment (ROI) while transitioning into AI may be uncertain. Even though AI technology has the potential to boost efficiency and reduce costs, the actual return on investment is vague. As Deloitte notes, calculating ROI for AI implementation and integration remains more art than science. Thus, predicting the financial benefits of adopting AI is challenging due to the complexity of implementation and high initial cost. Consequently, the time factor required to realize these benefits, and the possibility that the adopted AI system might not perform as expected, will likely lead to a lower-than-expected ROI. The long-term nature of AI investments means returns can be gradual and unpredictable,[17] increasing the risk that businesses might not achieve the anticipated financial gains, potentially impacting both profitability and stability of the organization.
Market volatility is another aspect to consider as AI adoption becomes mainstream, and AI tools are increasingly being relied on. This “herd mentality” could create shocks to markets, further deteriorating the stability and profitability prospects.[18] The economic environment can influence both costs associated with AI adoption and the potential returns. Additionally, sudden changes in market dynamics, such as new regulations, shifts in consumer behavior, or economic downturns and/or geopolitical events, can impact the effectiveness and profitability of AI investments. Hence, the rising AI adoption trend among businesses will likely create pressure on the global market and has the potential to impact business stability and profitability.
Thus, adoption of AI-inclusive business processes must include financial considerations related to costs, uncertainty of ROI and market volatility to maintain a stable business environment and steady revenue flow. Considering the financial aspects implementing or integrating AI can be costly and presents financial risks to business operations and stability and profitability of the organization, since it might divert business attention from other strategic goals.
- Human capital risks for companies adopting AI technology include talent shortages, resistance to AI, and the need for extensive training.
The talent shortage threatens to undermine the efficiency of AI transition. The lack of available talent not only delays AI projects but also pushes companies to compete aggressively for the few qualified professionals,[19] leading businesses to rely on external consultants. This, however, may create short-term relief, yet in the long term there are risks of eroding the company’s internal capabilities and strategic autonomy. Therefore, the lack of skilled AI professionals will likely lead to a more aggressive approach to talent acquisition to provide immediate relief. Looking forward, organizations will likely face erosion of its internal capabilities and strategic autonomy, impacting business operations.
Resistance to AI or resistance to change will likely increase the lack of trained employees, both of which can destabilize the business operations and the financial outlook. There is a potential that employees could breed doubts and resist the adoption of AI.[20] Moreover, this technological shift occurs at an accelerated pace, threatening to render certain jobs obsolete in just a decade. Workforce may fear job loss[21] or struggle to adapt to modern technologies, leading to decreased morale and productivity. Therefore, resistance to AI threatens to negatively impact operational and financial aspects of businesses, as the technological shift will likely replace certain jobs, as well as exert pressure on employees who are struggling to adapt to this trend.
How can we help?
Intelligence Solutions
The combination of business, market and strategic intelligence ensures result-driven outcomes for our customers.
Risk management
Risk management through the responsibility of taking risk ownership while ensuring safety and security
Training programs can quickly become outdated, because of the rising growth of AI innovation and the scarcity of AI-skilled professionals. Additionally, the considerable time and financial resources required for effective training may strain organizations, especially if trained employees leave for more competitive offers, resulting in a loss of investment.[22] Moreover, inadequate or poorly designed training programs can lead to a superficial understanding of AI, increasing the risk of errors, inefficiencies, and even ethical lapses, which could compromise the integrity and success of AI initiatives. Hence, talent shortage, resistance to AI and poorly designed training programs are vital risk considerations for companies aiming to adopt AI solution into business operations.
- Ethical risks of AI integration in businesses, which can impact stability and profitability, include data privacy concerns and transparency issues.
Besides the already mentioned algorithmic bias as an operational risk in AI adoption, few other ethical aspects are worth examining and are considered as a fundamental component of responsible business practices.
Data privacy is one of the most important aspects in AI implementation. AI systems require access to large amounts of data, as well as sensitive customer information. Improper management and security of data leads to breaches, erodes customer trust, and additionally exposes the organizations to costly legal procedures and regulatory fines. The likely result is a negative impact on financial stability. Furthermore, compromised data can lead to a damaged reputation and lost market share. In fact, the financial burden of rectifying a data breach and managing its fallout can undermine profitability and stability. Hence, data privacy is a fundamental concern for organizations transitioning to AI-inclusive processes. The companies that implement proper management and security of data will maintain client trust, and maintain business stability and profitability from financial burdens, eroded reputation and lost market share.
AI Transparency is crucial for maintaining business integrity. The opacity of AI systems creates difficulties for understanding the decision-making process or holding the AI systems accountable, which can result in customer distrust, legal issues and loss of credibility. Failure to explain the AI-based decisions (where AI is entrusted to do the decision-making) will eventually lead to customer attrition, eroded employee morale and ultimately business profitability. Therefore, ensuring transparency and accountability in implemented AI systems is critical to maintaining trust, and warrants that the technology aligns with ethical standards and business goals.
Thus, organizations that prioritize proper data management, data security and maintain transparency in AI-based operations will likely maintain stability of business operations and revenue generation.
03
Regulatory Compliance in AI Domination
03
Regulatory Compliance in AI Domination
Regulatory compliance of AI is evolving rapidly, and various regions are taking steps to address the risks associated with it. The EU, UK and US are a step ahead in regulating the development and use of AI.
EU’s AI Act is a groundbreaking legal framework in Europe that aims to foster trustworthy AI. The Act establishes governance structures at both European and national levels. It provides stricter regulations with clear requirements and obligations for AI providers and deployers while minimizing administrative burdens for businesses, especially small and medium enterprises.[23] So, providers and deployers of high-risk AI systems have specific obligations, including maintaining documentation, monitoring performance, and addressing safety concerns. Also, the Act covers high-risk AI applications, such as those used in critical infrastructures and safety components of products. Moreover, the Act prohibits certain AI practices that pose risks that could lead to discrimination or harm individuals.
Download Report
The Transformative Effects of AI on Business Operations
AI implementation enhances business efficiency, accuracy, and decision-making, leading to improved overall performance and competitive advantage.
Furthermore, the Act emphasizes transparency and explainability. Therefore, users should know when they are interacting with an AI system, and decisions made by AI should be reasonable. Before deploying high-risk AI systems, providers must undergo a conformity assessment to ensure compliance with safety and ethical standards.
To oversee the AI Act enforcement and implementation, the EU Commission in February 2024 established the European AI Office which will oversee the implementation and enforcement of the regulation. Besides, it collaborates with national authorities to oversee compliance and address any violations. Lastly, the European AI Office strives to position Europe as a leader in ethical and sustainable AI. Additionally, the European Commission has initiated a consultation process for a Code of Practice specifically for providers of general-purpose AI (GPAI) models.[24] This Code, expected to be finalized by April 2025,[25] will address critical issues such as transparency, copyright compliance, and risk management, and it will play a crucial role in shaping the operations of GPAI providers within the EU.
The UK Government has adopted an outcome-based framework for regulating AI aiming to create an equilibrium between innovations and risks mitigation.[26] The framework is not codified into law immediately since it needs time for regulating AI and putting it in practice. Hence, the framework focuses on five core principles: safety, security and robustness, appropriate transparency and explainability, fairness, accountability and governance, and contestability and redress. In anticipation of future needs, the government plans to introduce specific legislative measures. For that reason, these interventions aim to fill gaps in the existing regulatory framework. Higher priority is given to the risks associated with complex General Purpose AI and the foremost investors driving its development. So, organizations and companies should be ready for intensified and be ready to adjust for the upcoming AI regulatory activity in the coming year, which will involve guidelines, data collection, and enforcement.
To govern the AI regulations, the UK established Central Function, which will facilitate coordination among regulators and prevent divergent interpretations. This clarity will assist businesses in adopting and scaling AI investments, thereby enhancing the UK’s competitive edge.
The US regulatory framework for AI has recently evolved significantly, driven by the Executive Order on the Safe, Secure, and Trustworthy Development and Use of Artificial Intelligence, which was issued in October 2023.[27] The National Institute of Standards and Technology (NIST) has been central in this effort, producing critical documents and frameworks to support AI developers and users in managing AI-related risks. The NIST issued three final guidance documents, including one from the US AI Safety Institute, which helps AI developers evaluate and mitigate risks associated with generative AI and dual-use foundation models.[28] Additionally, NIST released a software package to measure how adversarial attacks impact AI system performance.[29] These new guidelines for risk mitigation associated with AI technology are the following:
- Artificial Intelligence Risk Management Framework: GenAI Profile (NIST AI 600-1).
- Secure Software Development Practices for GenAI and Dual-Use Foundation Models (NIST SP 800-218A).
- Global Collaboration on AI Standards (NIST AI 100-5).
- Managing Misuse Risk for Dual-Use Foundation Models (NIST AI 800-1).
Furthermore, the US’ Executive Order fosters AI innovation and competition by backing initiatives like the National AI Research Resource program and supporting small businesses. Also, it prioritizes consumer privacy through transparency, privacy-enhancing technologies, and compliance with federal nondiscrimination laws. Additionally, the Order tackles algorithmic bias, aiming to promote equity and civil rights across AI-impacted sectors.
Regulating AI encounters challenges like Internet regulation, including sovereignty, jurisdiction, and domain issues. Transparency and harmonized regulations offer partial solutions. The rapid evolution of AI poses a challenge, emphasizing the need for regulations to evolve as well, requiring continuous vigilance.
04
Over-Reliance on External AI Providers
04
Over-Reliance on External AI Providers
Relying on external AI providers introduces risks such as vendor lock-in, innovation dependency, intellectual property issues, service interruptions, data ownership and security concerns, and potential shifts in the vendor’s business model.
Risk | Risk Factors | Mitigation |
Vendor Lock-In | Over-reliance on a single AI provider, rapid pace of technological advancements, difficulties switching vendors | Vendor assessment, outsourced entities assessment, evaluation of data practices, security measures and compliance with regulations, media monitoring and analysis |
Innovation Dependency | Dependency on vendor innovation cycles, limited flexibility | Vendor assessment, outsourced entities assessment, market standing, media monitoring and analysis |
Service Interruptions | AI service outages, technical issues, connectivity, and unreliable vendor performance | Vendor assessment, continuous monitoring of performance and impact of AI systems |
Intellectual Property Risks | Loss of control and unauthorized use of data, and ownership of AI outputs | Identify and evaluate IP infringement, vendor assessment, competitor and market analysis |
Data Security and Sovereignty Risks | Data breaches, loss of data ownership, cybersecurity attacks and vendor data misuse | Vendor assessment, identify and evaluate risks, develop mitigation strategies, continuous monitoring, market analysis, assess international competition, assess geopolitical considerations related to cross-border data flows, cloud services and global supply chains |
Vendor Business Model Shifts | Changes in vendor pricing, service quality, and strategic realignment | Vendor assessment, enhanced due diligence, evaluate vendor’s financial stability, assess vendors’ market strategy, market analysis |
Vendor lock-in occurs when companies over-rely on specific third-party AI provider making it costly or complex to switch providers or adapt to new technological advancements. The primary concern with AI vendor lock-in is the rapid pace of technological change.[30] This dynamic environment raises the risk that a company could be “locked” into a long-term contract with a provider whose technology becomes obsolete or inferior to emerging solutions. Organizations might find themselves constrained by proprietary systems, data formats, or integration processes that are not easily transferable, creating barriers to leveraging newer, more advanced AI technologies.
Innovation dependency refers to the reliance on an AI vendor’s ability to continually innovate and keep their technology ahead of the curve. Companies solely relying on third-party vendors will likely become passive consumers, rather than active inventors. Such dynamics influence not only the company’s competitive position but also its operational resilience and strategic flexibility. Innovation dependency is closely tied to the dynamics of vendor lock-in but goes further by emphasizing the reliance on a vendor’s capacity to sustain technological leadership. When a company relies heavily on an external AI provider, its ability to adopt the latest advancements is contingent on the provider’s commitment and capability to innovate.[31] This dependency can create vulnerabilities if the vendor’s innovation pace slows, if they are overtaken by competitors, or if their strategic focus shifts away from areas critical to the business. Furthermore, this dependency can lead to stagnation, where a company’s operational capabilities are capped by the limitations of the AI provider’s technology.
Service interruptions are also a critical aspect related to the potential instability of AI vendors that adds another layer of risk. If the third-party provider experiences downtime or service disruptions, the impact will likely be felt on the business operations. As AI becomes increasingly embedded in business operations, the consequences of service disruptions can extend beyond immediate operational setbacks, affecting customer satisfaction, compliance with service level agreements, and overall business continuity.[32]
Additionally, any technical issue can result in service interruptions. The risk is amplified in cases where AI services are delivered via cloud-based platforms, which are vulnerable to connectivity issues, server failures, or regional outages affecting the provider’s data centers. Moreover, cybersecurity incidents can disrupt vendor’s services, compromise sensitive data, and require extensive downtime to resolve.
How can we help?
Intelligence Solutions
The combination of business, market and strategic intelligence ensures result-driven outcomes for our customers.
Risk management
Risk management through the responsibility of taking risk ownership while ensuring safety and security
Strategic Advisory
The first step in protecting your organization, assets and people is the identification of the risks and threats.
Cybersecurity incidents introduce a geopolitical dimension as well, since state-sponsored cyber activities[33] are on the rise, and AI systems are being prime targets due to their critical role in business operations. The interconnected nature of AI systems means that a breach or attack on one component can have cascading effects across the entire service, further amplifying the disruption. Also, vendor-specific issues, such as poor service management, lack of adequate support, or operational challenges like financial instability or changes in strategic direction, can also lead to service disruptions. Hence, companies reliant on a single AI vendor could find themselves vulnerable if that provider faces internal disruptions, strategic shifts, or fails to keep pace with the market.
Intellectual property concerns are a major issue for businesses transitioning to external provider business model. Engaging with AI vendors involves the use of proprietary algorithms, data sets, and models that can create complexities around ownership, usage rights, and the protection of sensitive information. One of the primary IP risks is the potential loss of control over data and outputs generated by the AI systems. In addition, when businesses share their proprietary data with third-party vendors, they may inadvertently expose themselves to IP infringement,[34] and to other related risks such as data misuse or unauthorized data replication. Another concern is the ownership of AI-generated outputs. In collaborations with AI vendors, questions about who owns the rights to the innovations or products developed using the AI tools will likely arise. If the AI solution contributes to the creation of new intellectual property, such as product designs, processes, or content, businesses need clear contractual terms to establish ownership and usage rights. Without these safeguards, companies might find themselves in disputes over the commercialization or exploitation of their AI-generated innovations, potentially losing out on the strategic benefits of their investments.
Data security and sovereignty are paramount. Data security concerns primarily revolve around the risk of unauthorized access, data breaches, and cyberattacks. Collaborating with an external AI provider involves sharing sensitive business data, which exposes the company to cybersecurity threats and data breaches.[35] These risks are particularly acute when dealing with AI models that require continuous data input, such as machine learning and deep learning applications. Data sovereignty is another key concern. When data is shared with an AI provider, questions arise about who owns the data, how it can be used, and whether the third-party provider has any rights to the insights or outputs derived from that data. Also, data sovereignty is entwined with broader strategic rivalries,[36] where data control has become a focal point of this technological decoupling. For organizations, data sovereignty introduces considerations affecting cross-border data flows, cloud services and global supply chains. Hence, without clear agreements, organizations may find that they do not fully control the use of their data, or worse, that the AI provider can use it to train other models or for purposes beyond the original scope of the contract.[37] Besides, this dynamic has the potential to lead to competitive disadvantages, especially if the provider preserves leverage the business’s data to benefit other clients, including competitors.
Shifts in vendor’s business model will likely introduce risks related to business continuity, cost, and alignment with strategic objectives. As AI vendors evolve their business models in response to market pressures, technological advancements, or financial considerations, their customers can find themselves facing unexpected changes that can disrupt operations and strategic plans.[38] One primary risk associated with shifts in a vendor’s business model is the alteration of pricing structures. In cases when the vendor scales or pivots towards profitability, there could be a shift to higher fees, tiered pricing, or additional costs for access to advanced features and support. This will likely lead to budgetary challenges for businesses that have integrated the AI services into their operations, making it difficult to absorb sudden cost increases or switch to alternative solutions without significant disruption.
05
End notes
05
End notes
Transitioning into AI business processes introduces substantial risks, including implementational, operational, financial, human capital, and ethical risks. Geopolitics and regulatory compliance are also a moving target as governments worldwide strive to oversee AI technologies effectively.
Mismanaging these risks can result in economic losses, reputational damage, and legal repercussions, potentially outweighing the benefits of AI adoption. For companies transitioning to AI-inclusive business models, a comprehensive risk management strategy is essential. This involves not only a technical understanding of AI systems but also a holistic approach to integrating AI responsibly and sustainably into the organization’s operations. Dynamics International Group offers specialized consultancy services to guide businesses through the complexities of AI adoption, emphasizing a balanced approach that maximizes value while mitigating risks. Our expertise in risk assessment, enhanced due diligence, and geopolitical analysis ensures that companies can leverage AI technologies with confidence.
From identifying and evaluating risks, conducting market analysis, developing mitigation strategies, implementing governance frameworks, continuous monitoring, documenting and reviewing, to ensuring regulatory compliance, Dynamics International Group is your partner in navigating the AI ecosystem. Our tailored solutions help clients capitalize on AI innovations, drive growth, and gain a competitive advantage while safeguarding their assets against potential dangers.
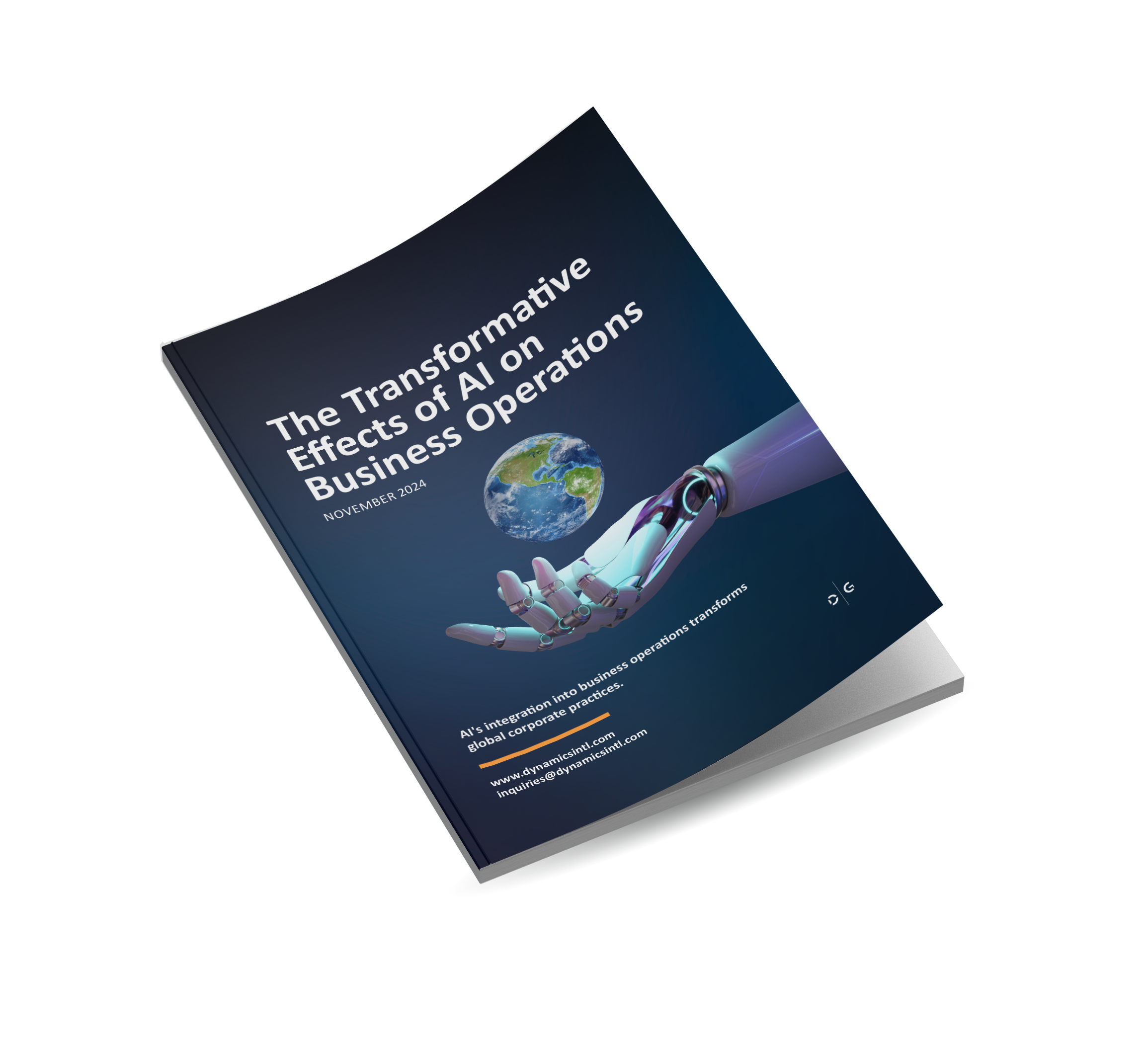
ARTICLE | 28 PAGES